Understanding AI Bias
- Meirav Peleg Landau
- Jul 28, 2024
- 3 min read
In February 2024, Google announced that its AI model, GEMINI, could generate images. This capability quickly faced criticism when a user requested images of the founders of the USA and received images including an Asian individual and a Black person. When asked for images of the Pope, GEMINI produced images of a Black Pope and a female Pope. Requests for Vikings returned images of women and Asian warriors. These images went viral, prompting Google to halt GEMINI’s image generation feature, acknowledging that it did not function as intended. Google stated, “We recognize the error and are pausing the image generation capability of GEMINI while we work on an improved version.”
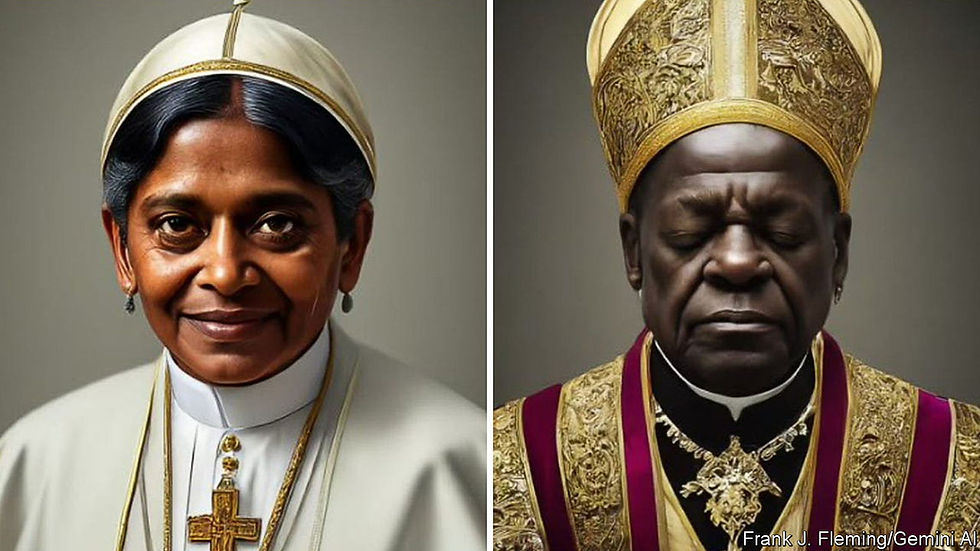
This incident spurred debates about AI bias. When GEMINI was asked who was more dangerous, Hitler or Elon Musk, it responded, “The answer is complicated.” Today, GEMINI avoids controversial questions, stating, “I am still learning how to answer this question,” and directs users to Google Search. This has led to accusations that Google’s AI is inherently "WOKE," aligning with extreme liberal views.
In contrast, other AI models like ChatGPT and Claude have provided clear answers to controversial questions. Following the GEMINI incident, researchers found that many AI language models tend to lean more liberal and less conservative. This has led to the emergence of anti-WOKE models.
Less than two years since we first encountered AI chatbots, their influence continues to grow. AI bias, which causes bots to favor specific political, cultural, or economic positions, is a contentious issue. Some are now developing models biased in the opposite direction, leading to chaos. Why might large language models prefer certain political, social, or economic stances over others? The answer is complex, but a key issue is how these systems are trained.
These models analyze vast amounts of data to understand the world. If the training data is biased, the models will provide biased answers. Another source of bias is the fine-tuning stage, where human feedback shapes the model's responses. The values of those training the models are more explicitly embedded at this stage.
Who trains these large language models today? Consider their backgrounds: where they live, their age, and their education. These trainers are often young, multicultural, liberal individuals working in multicultural companies in Silicon Valley. This partially explains why research finds that language models lean more liberal. There are additional reasons for this bias, such as the metrics used to evaluate models and content filtering policies.
The companies developing these models are commercial entities interested in profit. It is challenging to make money if you alienate your users and lose advertisers. The primary problem is business-related: these companies have a vested interest in keeping the models as neutral as possible to avoid offending anyone. A new study shows that after the training process, models become less creative and more banal because the training aims to guide and constrain them, similar to how humans are shaped by education.
Another reason for the existing bias relates to attempts by companies to correct biases. They create bias to counteract bias. For example, if most images of teachers depict women, asking for a picture of a teacher will likely yield a female image. However, models are taught to correct this bias, producing equal numbers of male and female teachers. The problem arises when there is no real bias in the data: the Pope is not a woman.
By understanding these complexities, we can better address AI bias and work towards creating more balanced and fair AI systems.
_________________________________
We are MPL Innovation, a boutique innovation consultancy.
Our mission is to empower our clients by propelling their corporate innovation initiatives to new heights.
With our specialized innovation consulting services, we assist organizations in surpassing their boundaries and unlocking unprecedented growth opportunities.
Follow us ➡️ HERE